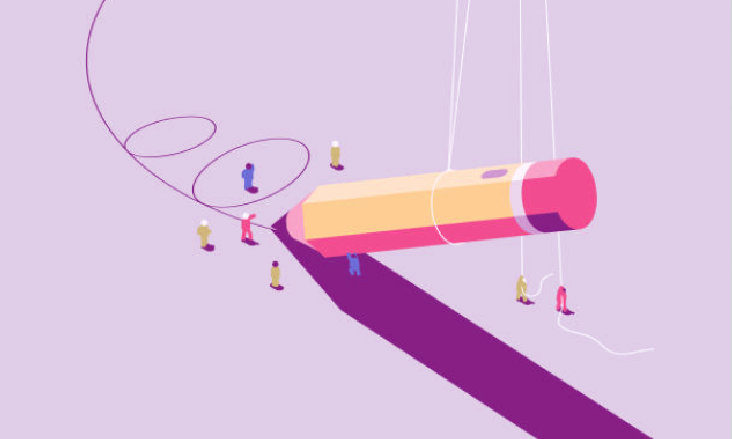
Data Science and Data Analytics - know How They are Different
Data Science and Data Analytics are the buzzwords in the job market today. But most of the people due to their sharing of similar word “data” often assume that these two fields are similar and interchangeable.
Little did they know, both the fields are completely different and have different attributes attached to them. So if you are someone who is constantly searching for “what is the difference between data science and data analytics with example” or “difference between Data Science and Data Analytics and Data Engineering”, in the following, you will find differing statements to explain these two disciplines.
What is the Difference Between Data Science and Data Analytics with Example
What is Data Science?
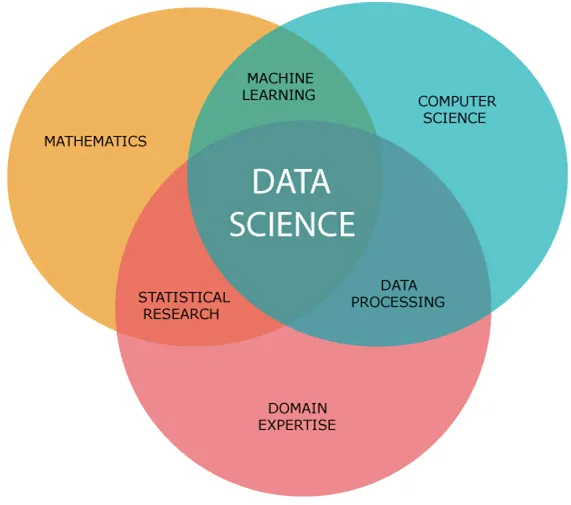
Data Science in simple words is a study of Data. The field requires developing methods to record, store, and analyze the data to retract useful information from that.
- Data Science is a process of gaining insights or valuable information on any type of given data, be it Structured Data or Unstructured Data.
- Data Science covers every type of data analysis, which might or might not require computer use, and it is more closely related to the mathematics field of statistics, which is inclusive of the collection, organization, analysis, and presentation of data.
Data Science has become an integral part of IT hubs of companies as it helps them to analyze and maintain the large amount of data produced for making critical decisions and actions.
For example - A company or organization that has petabytes of user data (1015 or 1,000,000,000,000,000 bytes) utilizes Data Science techniques and tools to store, analyze, and manage their data --- to extract great understandable insights or information from the data.
Brand Example - Uber Using Data Science For Reinventing Transportation
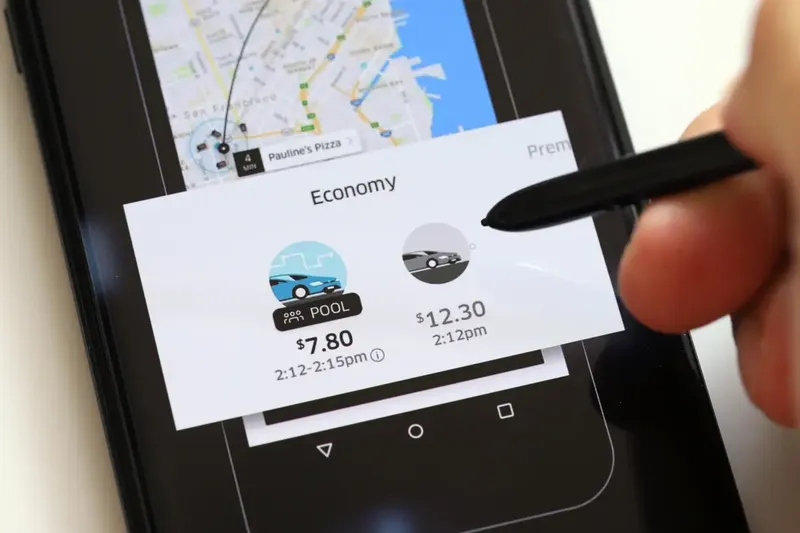
Uber, the renowned ride-sharing company leverages Data Science to gather and analyze the data of its customers and its drivers in an aggregated and anonymized form. To understand what features of the services were used the most, which gives the brand a scope to understand which area of service to focus on more to drive great value for the customers and its company.
The people who are behind analyzing and managing data are called Data Scientists. Data Scientist jobs are booming day-by-day. IBM predicts that there will be 364,000 jobs for Data Scientists in the USA in the future. Anyone can choose to enter this field by taking the accredited Data Science Training to gather the right skills and certification for the job market.
What is Data Analytics?
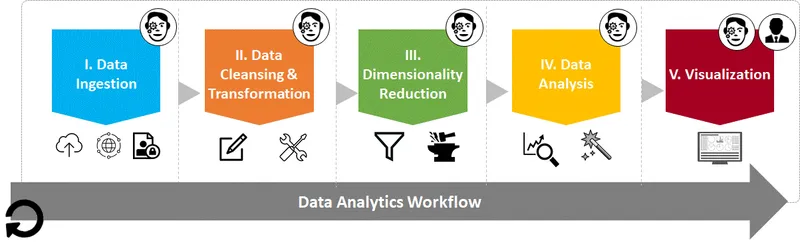
Data Analytics is the processing of datasets to draw concussions from datasets. It takes the raw data and extracts valuable insights from it.
- Data Analytics techniques leverage specialized software and systems that integrate the capabilities of the machine learning algorithms, automation, and more.
- Data Analytics helps businesses better understand their customers, stakeholders, evaluate the ad campaign, create content strategies with personalized content formation, and improve and develop products as per customer’s preferences.
- Data Analytics helps businesses take important business decisions that could help improve their operations, products, and bottom line.
For example - There are three types of data a company inherits:
- First-party data - That is obtained from customers.
- Second-party data - That is obtained from the known organizations that collected it.
- Third-party data - That is obtained from the marketplace.
Businesses use historical data or new data to drive their analytics. The data can be purchased as well from organizations that are into collecting data.
Brand Example - Coca Cola Using Data Analytics for Customer Positioning
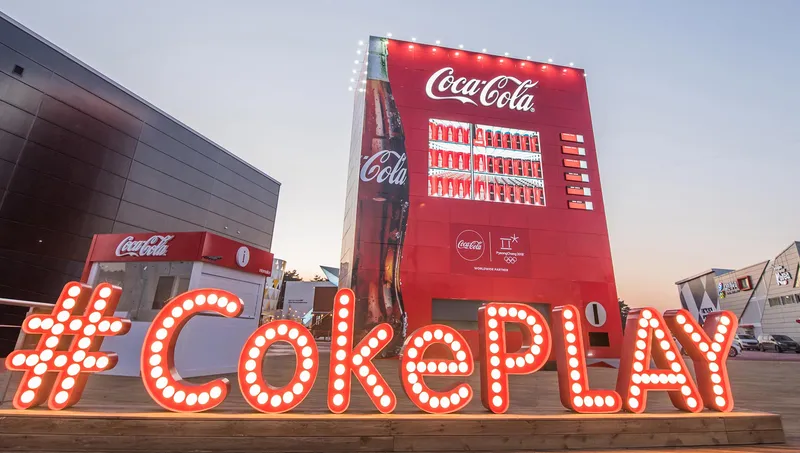
The coca-cola brand by using AI and Data Analytics techniques draws customers data from its vending machines. This act is helping businesses understand what flavor of drink the customers are preferring the most. This monitoring of the flow of data helped the brand to release the Sprite Cherry flavor in certain markets.
Both the Fields are into Data Transformation so what’s the difference between Data Science and Data Analytics?
Data Science is an umbrella term, while Data Analytics is a more focused version of this and can be considered a part of this larger process.
Data Science being a broader term requires to prepare the questions around the datasets, while the Data Analysts are further required to respond and process these prepared and asked questions.
What is the Difference Between Data Science and Data Analytics and Data Engineering?
Data Science
Data Science is a process of analyzing and managing complex datasets and preparing questions based on them.
Data Analytics
Data Analytics is a process to transform the numeric data by answering the questions prepared based on them to help businesses make better decisions.
Data Engineering
Data Engineering is the process of preparing the data by focusing on the practical applications of data collection and analysis.
Skills/Qualification Required to Become Data Scientists and Data Analysts
Data Scientists
- Knowledge of database systems like MySQL, Hive, etc.
- To be well-versed with Python, R, MapReduce job development.
- To know of median, rank, and their use on datasets.
- To know of deep analytics insights, machine learning, clustering, etc.
- To know the implementation of maths, stats, correlation, data mining, and predictive analysis to drive better predictions and queries for decision making.
Data Analytics
- Knowledge of SQL and data analytics along with data warehousing and BI concepts.
- To be well-versed with Hadoop based analytics.
- To know of data retrieving tools and skills.
- To know of data architecture tools and components. And should have proficiency in decision making.
- To know of ETL tools, to transform data stores into analytics data stores.
Final Thoughts on What is the difference between Data Science and Data Analytics with Example!
Hope the above guide answers your query on “What’s the difference between Data Science and Data Analysts” along with the “difference between Data Science and Data Analytics and Data Engineering” with detailed explanation and range on examples based on brands like Coca-Cola and Uber.
Data Science and Data Analytics are the two buzzwords of the job markets that due to their commonality of the word “Data” are inferred as the same and interchangeable. Whereas, in reality, the two terms have the same role to gather, analyze, and manage the data, but data science is considered to be an umbrella term that involves generating questions to be answered around datasets, whereas data analytics requires answering of those prepared questions with accurate methods and procedures.
Data Science is a promising career today one can think of absorbing in by taking the effective and real-time projects-based Data Science Certification Training.