How you can use data lakes to effectively drive your marketing campaigns
With no re-engineering, you can now prepare the lake to support a marketing campaign on criteria for which you had the data, but you had not anticipated the questions to ask of the data or the model to support them.
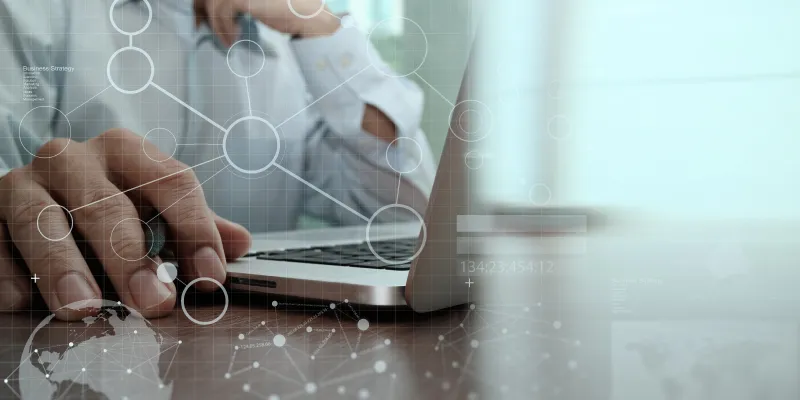
Data lakes are touted as a more flexible alternative to data warehouses, seeing that they are faster to implement, gathering all of an organisation’s data relevant for analysis on a given domain.
This is specifically relevant in the marketing domain: a data lake is the best approach to quickly turn an ever-changing collection of potentially large datasets about customers and their interactions with your company into insights that sales teams need. They allow for shorter time to value thanks to just-in-time exploration and modeling on data pre-populated in the lake, made possible by data discovery and data integration tools now available on big data environments.
The value of “schema on read”
But first, some clarification. Data mart is a commonly used term, referring to a subset of a data warehouse that usually has an enterprise-wide depth. To quote James Dixon from his original post announcing data lakes:
“If you think of a data mart as a store of bottled water – cleansed and packaged and structured for easy consumption – the data lake is a large body of water in a more natural state. The contents of the data lake stream in from a source to fill the lake, and various users of the lake can come to examine, dive in, or take samples.”
The primary motivation of creating data lakes was to maintain any data that may be relevant for analysis on a given domain, now or in the future. After you ingest diverse datasets in raw form they are not directly consumable, but the idea is that you’ll defer this activity until you explore and discover a subset of the lake worth analysing to validate a business hypothesis about the data. Once this is validated, you can prepare and model this data lake subset in a data schema to support more complete analysis.
This “schema on read”, which puts off preparation and modeling until you find a business reason for using the data, is the game changer. With no re-engineering, you can now prepare the lake to support a marketing campaign on criteria for which you had the data, but you had not anticipated the questions to ask of the data or the model to support them. Compare this with a traditional data warehouse and its “schema-on-write” approach (i.e., upfront modeling and conformance of the ingested data to the model): you are sent back to the drawing board when a new, unanticipated dataset needs to be added to the mix.
Drivers for the multiplicity of disparate data in marketing
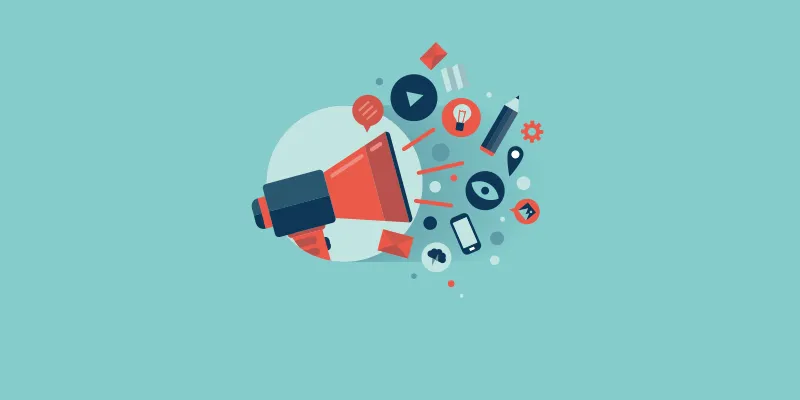
But what is it about marketing that these “unanticipated datasets” turn up so frequently? Well, as a marketer, you listen to what your customers are telling you, through the data you collect about them and their interactions with you. What they care about, what they respond to and what they disregard. It’s then up to you to contact them with content that’s of interest, spending your budget for maximum impact.
So, beyond your company’s internal databases storing prospect data, customer data and transaction data, you also need data about the interaction points, and whatever additional data you can get about your potential buyers. For example, you can associate channels to each interaction, and get data from data sources covering those channels: opened email, what they search for online, pages visited on your website, content viewed, their social media profile and level of influence, attendance to events (in B2B) as well as purchased data such as basic demographics data (based on a user’s IP addresses in B2C, or postal addresses in B2B), credit ratings etc.
These datasets come in many formats and types. Their number tends to increase because they reflect interaction points made possible by new technology, new business models and new market players. Say a new interaction point, e.g., smartphones, is introduced and managed with a new marketing automation tool. You’d like to ingest the data as soon as possible, have it explored by data scientists and integrate it with the rest when exploration and early analysis shows, for instance, the high correlation between people reacting to the smartphone campaign and those clicking on certain kinds of ads. This is faster to do with a data lake than with a data warehouse.
The need for just-in-time integration in the marketing data lake
Now let’s consider the customer journey, which is becoming increasing complex. As an example, you want to buy a sound docking station. You watch a demonstration on YouTube and see a brand that interests you. You visit the brand’s vendor and read a blog post about the sound quality of the technology. Later, you’re served up display ads for that brand while visiting a media site. You click on the ad, go to the vendor’s site, fill in a form and download a buyer’s guide. The next day, as you pass by a store, a coupon appears on your smartphone, enticing you to purchase the docking station. You hear the product’s sound and decide to purchase.
In this scenario, you have used numerous channels, each time moving closer to conversion. Recent data shows that depending on the industry, it takes between three and seven interactions for a B2C lead to convert (B2B leads take fewer interactions per person, but purchases are made by buying teams). Each interaction builds up on the other, so attributing a conversion to a single interaction (say, the mobile coupon) doesn’t make sense. Yet it’s crucial to associate revenue with marketing spend to determine the most effective marketing channels to use in your next campaign and to improve marketing accountability.
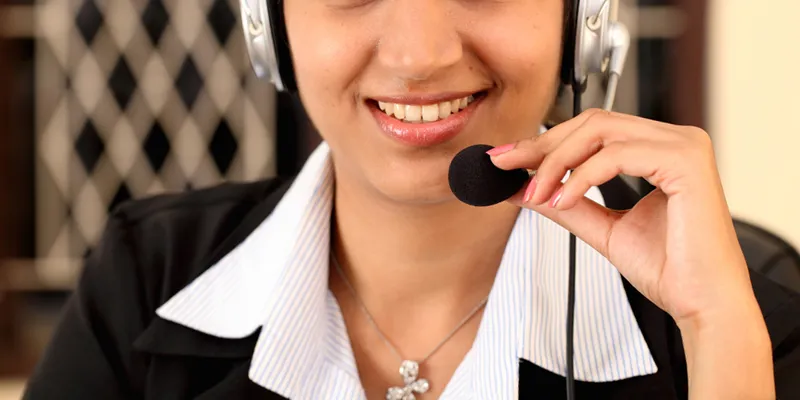
In addition, the more data you can attach to customers, the more you will know what drives them to buy and how best to personalise the content you send them.
This is why integrating your customer interactions - by marketing application tools, CRM data, website activity from known visitors, social media data about prospects, as well as transactions for existing customers - allows you to understand customer journeys more fully. This in turn leads to improved attribute revenue, qualified leads and “nurture” leads by sending the right content.
Democratising just-in-time integration
One final thought – how to allow teams of users beyond IT, such as data scientists and business analysts, also work with this data? Data lakes now come with data integration toolsets that can be used by these non-IT audiences. Traditional data integration tools are still needed, especially for IT developers to productise a data model from a collection of prepared data sets produced by data scientists and analysts. Allowing these different user populations to work collaboratively will go a long way in creating even more value around the use of data lakes.
(Disclaimer: The views and opinions expressed in this article are those of the author and do not necessarily reflect the views of YourStory.)